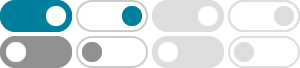
Welcome to Yuhong Yang's Homepage - College of Liberal Arts
Yuhong Yang Professor, School of Statistics - University of Minnesota. Curriculum Vitae Office hours (Zoom): Papers and Manuscripts Research Interests: Nonparametric function estimation. High-dimensional data analysis. Model selection/combining in both theory and application.
Chenglong Ye, Yi Yang and Yuhong Yang (2018). Sparsity oriented importance learning for high-dimensional linear regression, Journal of American Statistical Association, Vol. 113, 1797-1812.
Yang Yuhong - Papers - College of Liberal Arts
Guowu Yang and Yuhong Yang (2019). Minimax-Rate Adaptive Nonparametric Regression with Unknown Correlations of Errors. SCIENCE CHINA Mathematics , vol. 62, 227-244.
Statistics 3021: Introduction to Probability and Statistics
Statistics 3021, Spring 2013 . Class Hours: Lecture: MWF, 11:15 - 12:05 in AkerH 209 . Instructor: Yuhong Yang . 376 Ford Hall . Email: [email protected]; Phone: 612 ...
Statistics 3021: Introduction to Probability and Statistics
Statistics 3021, Spring 2016 . Class Hours: Lecture: MWF, 11:15 - 12:05 in 16 Vincent Hall . Instructor: Yuhong Yang . 376 Ford Hall . Email: [email protected] ...
data-dependent and adaptive (see, e.g., [7,31,34,39,57,58,70]). Yang [70] showed that the asymptotic optimality of BIC for a parametric scenario (which follows directly from consistency of BIC) and asymptotic optimality of AIC for a nonparametric scenario can be shared by an adaptive model se-lection criterion.
4 D. FERRARI AND Y. YANG where L q(u) = logu if q = 1 and L q(u) = (u1−q −1)/(1−q) otherwise. The function L q represents a Box-Cox transformation in statistics and in other contexts it is often called a deformed logarithm. Note that if q → 1, then L q(u) → log(u) and the usual definition of Shannon entropy is recovered.
Yuhong Yang School of Statistics University of Minnesota Minneapolis, MN 55455 Abstract While there are various model selection methods, an unanswered but important question is how to select one of them for data at hand. The difficulty is due to that the targeted behaviors of the model selection procedures
simplified density estimation context, Yang (2001c) showed theoretically the advantage of a proper com-bining over any selection method. In this work, we propose the use of a method, named AFTER, to combine the forecasts from the individual candidate models. The idea is that, with an appropriate weighting scheme, the combined forecast
Yang (2003) showed empirically that when AIC and BIC estimators are properly combined, the new estimator tends to perform like the better one under the squared error loss.