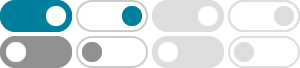
Title: You Only Look Once: Unified, Real-Time Object Detection
Jun 8, 2015 · We present YOLO, a new approach to object detection. Prior work on object detection repurposes classifiers to perform detection. Instead, we frame object detection as a regression problem to spatially separated bounding boxes and associated class probabilities.
YOLO Explained: From v1 to v11 - viso.ai
Dec 6, 2024 · The YOLO approach is to apply a single convolutional neural network (CNN) to the full image. This network divides the image into regions and predicts bounding boxes and probabilities for each region. These bounding boxes are weighted by the predicted probabilities.
YOLOv1 Explained - Papers With Code
YOLOv1 is a single-stage object detection model. Object detection is framed as a regression problem to spatially separated bounding boxes and associated class probabilities. A single neural network predicts bounding boxes and class probabilities directly …
YOLO v1 : Part 1. YOLO, short for You Only Look Once is a
May 4, 2018 · YOLO, short for You Only Look Once is a convolutional neural network architecture designed for the purpose of object detection. There are 3 versions of YOLO namely version 1, version 2 and...
YOLO-v1 to YOLO-v8, the Rise of YOLO and Its Complementary
Jun 23, 2023 · Next, the evolution of YOLO variants is presented, detailing the key contributions from YOLO-v1 to YOLO-v8, followed by a review of the literature focused on YOLO-based implementation of industrial surface defect detection.
GitHub - tanjeffreyz/yolo-v1: PyTorch implementation of the …
PyTorch implementation of the YOLO architecture presented in "You Only Look Once: Unified, Real-Time Object Detection" by Joseph Redmon, Santosh Divvala, Ross Girshick, Ali Farhadi
YOLO V1- An Intuitive Guide - Medium
May 16, 2020 · YOLO stands for You Only Look Once. It signifies that unlike sliding a feature extractor on the image multiple times, the algorithm looks at the image only once for detecting objects in it....
look once (YOLO) at an image to predict what objects are present and where they are. YOLO is refreshingly simple: see Figure1. A sin-gle convolutional network simultaneously predicts multi-ple bounding boxes and class probabilities for those boxes. YOLO trains on full images and directly optimizes detec-tion performance.
yolo-v1.ipynb - Colab - Google Colab
pred_areas = pred_wh[..., 0] * pred_wh[..., 1] true_areas = true_wh[..., 0] * true_wh[..., 1] # Calculate union areas and IoU scores union_areas = pred_areas + true_areas - inters ect_areas...
YOLO Explained: From v1 to v11 - Capa Learning
Dec 9, 2024 · The essence of YOLO models is treating object detection as a regression problem. The YOLO approach involves applying a single convolutional neural network (CNN) to the entire image. This network divides the image into regions, predicting bounding boxes and probabilities for …
- Some results have been removed