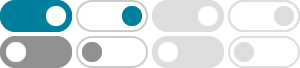
[2402.09329] YOLOv8-AM: YOLOv8 Based on Effective Attention …
Feb 14, 2024 · Experimental results demonstrate that the mean Average Precision at IoU 50 (mAP 50) of the YOLOv8-AM model based on ResBlock + CBAM (ResCBAM) increased from 63.6% to 65.8%, which achieves the state-of-the-art (SOTA) performance. Conversely, YOLOv8-AM model incorporating GAM obtains the mAP 50 value of …
UC Irvine - Faculty Profile System
In Ham, Jennifer, Ulrich Kinzel, and David Tse-chien Pan. The Origins of German Self-Cultivation: Bildung and the Future of the Humanities (pp. 151-154). Oxford: Berghahn.
Chun-Tse Chien - CSAuthors
Mar 31, 2025 · Combined heart rate variability and dynamic measures for quantitatively characterizing the cardiac stress status during cycling exercise. Comput. Biol. Medicine, 2015.
Chun-Tse Chien 1, Rui-Yang Ju 2, Kuang-Yi Chou 3, and Jen-Shiun Chiang 1,* 1 Department of Electrical and Computer Engineering, Tamkang University, No.151, Yingzhuan Rd., Tamsui Dist., New Taipei City, 251301, Taiwan; [email protected] (Chun-Tse Chien)
Chun-Tse Chien (0009-0008-7549-4021) - ORCID
ORCID record for Chun-Tse Chien. ORCID provides an identifier for individuals to use with their name as they engage in research, scholarship, and innovation activities.
Chun-Tse Chien - LG Electronics North America | LinkedIn
View Chun-Tse Chien’s profile on LinkedIn, a professional community of 1 billion members.
- 500+ connections
- Location: LG Electronics North America
Chun-Tse CHIEN | Research profile
Chun-Tse CHIEN | Cited by 21 | | Read 1 publication | Contact Chun-Tse CHIEN
Tse Chien (@tsechienkoo) • Instagram photos and videos
420 Followers, 1,692 Following, 612 Posts - Tse Chien (@tsechienkoo) on Instagram: "Someone who loves nature, food, camping, photography, travelling, volunteering and creative/DIY stuff!"
Chun-Tse Chien | DeepAI
Read Chun-Tse Chien's latest research, browse their coauthor's research, and play around with their algorithms
YOLOv9 for fracture detection in pediatric wrist trauma X‐ray …
Jun 12, 2024 · Since attention mechanisms [14-18] have excellent results in enhancing the performance of neural network models, Chien et al. achieved the state-of-the-art (SOTA) performance by incorporating different attention mechanisms into the YOLOv8 model.