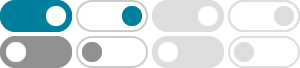
Autoregressive conditional heteroskedasticity - Wikipedia
Nonlinear Asymmetric GARCH (1,1) (NAGARCH) is a model with the specification: [6][7] where and , which ensures the non-negativity and stationarity of the variance process.
In this thesis, GARCH(1,1)-models for the analysis of nancial time series are investigated. First, su cient and necessary conditions will be given for the process to have a stationary solution. Then, asymptotic results for relevant estimators will …
Volatility Forecasting: GARCH (1,1) Model - Portfolio Optimizer
Mar 11, 2024 · In this blog post, I will detail the simplest but often very useful 4 GARCH (1,1) volatility forecasting model and I will illustrate its practical performances in the context of monthly volatility forecasting for various ETFs. This section contains reminders from a previous blog post.
(1−a − b,b,a) and the long run average variance is w /(1−a − b). It should be noted that this only works if a + b < 1, and only really makes sense if the weights are positive requiring abw>>>0,0,0. The GAR CH model that has been described is typically called the GARCH(1,1) model. The (1,1) in parentheses is a standard notation in which
We study in depth the properties of the GARCH(1,1) model and the assump-tions on the parameter space under which the process is stationary. In particular, we prove ergodicity and strong stationarity for the conditional variance (squared volatil-ity) of the process.
GARCH Model: Definition and Uses in Statistics - Investopedia
Oct 14, 2024 · GARCH is a statistical modeling technique used to help predict the volatility of returns on financial assets. GARCH is appropriate for time series data where the variance of the error term is...
11.1 ARCH/GARCH Models | STAT 510 - Statistics Online
A GARCH (generalized autoregressive conditionally heteroscedastic) model uses values of the past squared observations and past variances to model the variance at time \(t\). As an example, a GARCH(1,1) is \(\sigma^2_t = \alpha_0 + \alpha_1 y^2_{t-1} + \beta_1\sigma^2_{t-1}\)
10.2 Bollerslev’s GARCH Model | Introduction to ... - Bookdown
Hence, for many purposes the GARCH (1,1) model is the de facto volatility model of choice for daily returns. The statistical properties of the GARCH (1,1) model are derived in the same way as the properties of the ARCH (1) model and are summarized below: {Rt} {R t} is a covariance stationary and ergodic process provided α1 +β1 <1 α 1 + β 1 <1.
GARCH (1,1) - frds
The likelihood function for a GARCH(1,1) model is used for the estimation of parameters \(\mu\), \(\omega\), \(\alpha\), and \(\beta\). Given a time series of returns \(\{ r_1, r_2, \ldots, r_T \}\) , the likelihood function \(L(\mu, \omega, \alpha, \beta)\) can be written as:
Understanding the GARCH (1,1) model: the constant, the ARCH …
A GARCH(1,1) model is \begin{aligned} y_t &= \mu_t + u_t, \\ \mu_t &= \dots \text{(e.g. a constant or an ARMA equation without the term $u_t$)}, \\ u_t &= \sigma_t \varepsilon_t, \\ \sigma_t^2 &= \omega + \alpha_1 u_{t-1}^2 + \beta_1 \sigma_{t-1}^2, \\ \varepsilon_t &\sim i.i.d(0,1). \\ \end{aligned} The three components in the conditional ...
- Some results have been removed